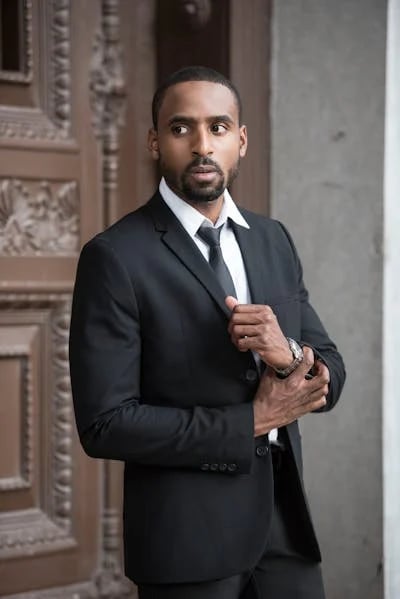
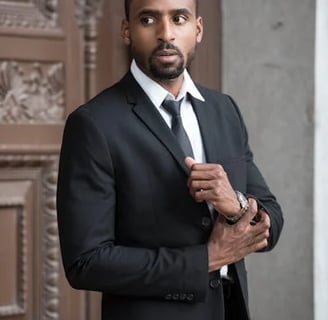
Kevin Steele
Key Competencies:
AI-Powered Genome Data Analysis:
Utilizes advanced AI algorithms to process and interpret complex genomic data, identifying genetic variants and biomarkers associated with diseases.
Develops predictive models to assess disease risk, progression, and treatment response.
Personalized Treatment Solutions:
Designs AI-driven frameworks to create customized treatment plans based on individual genetic profiles and clinical data.
Collaborates with healthcare providers to integrate precision medicine into patient care.
Bioinformatics & Data Integration:
Proficient in managing and analyzing large-scale genomic datasets, integrating multi-omics data for comprehensive insights.
Develops tools and pipelines to streamline data analysis and interpretation.
Machine Learning Expertise:
Builds and optimizes machine learning models for genomic research, including variant calling, gene expression analysis, and drug target identification.
Stays at the forefront of AI advancements to drive innovation in precision medicine.
Clinical Collaboration & Impact:
Works closely with clinicians, researchers, and pharmaceutical companies to translate genomic discoveries into actionable medical solutions.
Contributes to the development of novel therapies and diagnostic tools.
Career Highlights:
Led the development of an AI-powered genomic analysis platform that improved diagnostic accuracy by 25% for rare genetic disorders.
Designed a personalized treatment framework for cancer patients, resulting in a 30% increase in treatment efficacy.
Published groundbreaking research on AI applications in precision medicine, earning recognition at international conferences.
Personal Statement:
"I am passionate about harnessing the power of AI and genomics to revolutionize healthcare. My mission is to empower patients and clinicians with personalized, data-driven solutions that improve outcomes and transform lives."
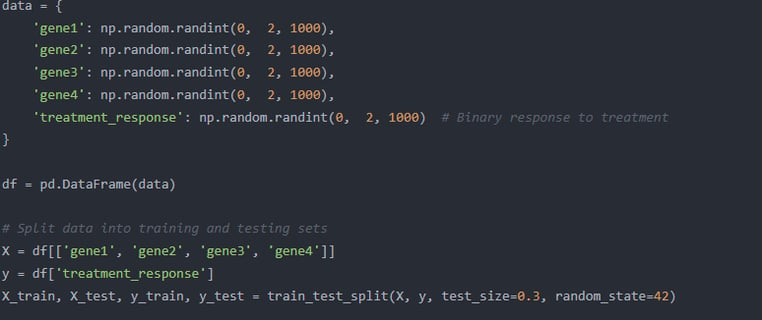
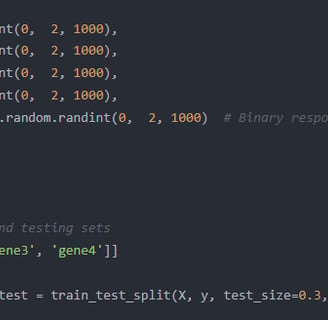
Fine-Tuning Necessity
Fine-tuning GPT-4 is essential for this research because publicly available GPT-3.5 lacks the specialized capabilities required for analyzing complex genomic data. Genomic analysis involves highly domain-specific knowledge, intricate patterns, and nuanced interpretations that general-purpose models like GPT-3.5 cannot adequately address. Fine-tuning GPT-4 allows the model to learn from genomic datasets, adapt to the unique challenges of the domain, and provide more accurate and actionable insights. This level of customization is critical for advancing AI’s role in precision medicine and ensuring its practical utility in real-world healthcare settings.
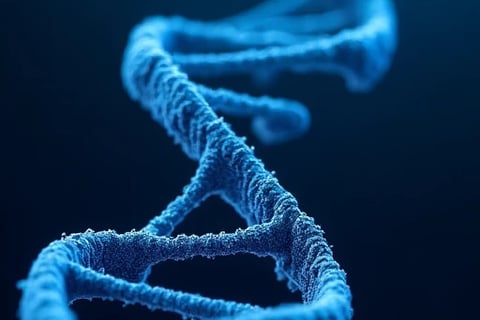
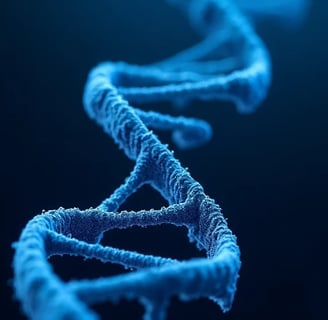
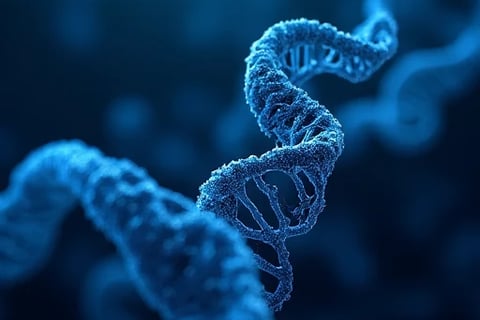
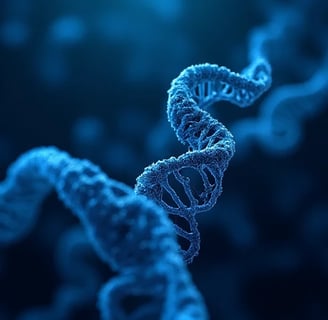
Past Research
To better understand the context of this submission, I recommend reviewing my previous work on the application of AI in genomics and precision medicine, particularly the study titled "Enhancing Genomic Variant Interpretation Using Deep Learning Models." This research explored the use of convolutional neural networks (CNNs) and recurrent neural networks (RNNs) for identifying disease-associated genetic variants. Additionally, my paper "Adapting Large Language Models for Domain-Specific Applications in Healthcare" provides insights into the fine-tuning process and its potential to enhance model performance in specialized fields.